THANK YOU FOR SUBSCRIBING

The AI Rat Race - Keeping Up with New Technologies or Waiting for Maturity?
Andreas Kurz, Global Head of Digital Transformation, ALFAGOMMA Group

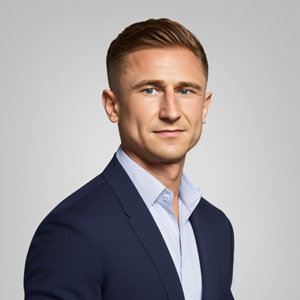
Andreas Kurz, Global Head of Digital Transformation, ALFAGOMMA Group
Andreas Kurz is the Global Head of Digital Transformation at Alfagomma Group. Based in Singapore, he leads cross-functional teams across Asia and Europe, driving AI adoption, cloud solutions and enterprise automation. With a strong background in both technology and strategy, Andreas focuses on turning emerging innovations into scalable business value.
Recognizing the author's deep, hands-on experience with early AI adoption and enterprise integration challenges, this exclusive feature delivers practical insights into managing the rapid evolution of AI technologies while building stable, business-driven solutions that deliver lasting value.
Artificial Intelligence (AI) has become the loudest, fastest-moving technology in the room. Every day brings announcements of game-changing models, frameworks, plugins and platforms, each promising to redefine how businesses operate. It feels like a rat race, not just for innovation, but for relevance, where speed of evolution often outpaces the ability to assess, implement and deliver value.
The pace of AI development is breathtaking: From basic chatbot interactions to multimodal reasoning, autonomous agent frameworks and real-time digital coworkers. Yet, alongside this velocity lies the inflationary bubble of hype. Every new release is labeled a breakthrough. Every vendor positions their product as essential. Demos and pilots abound, but few reach the rigor required for enterprise production.
-
The AI race isn’t about who implements the most tools or jumps on the latest trend. It’s about building lasting value with clarity and intent.
The truth is: while technology evolves fast, enterprise-grade deployments do not. According to a Forbes study, only one in four Minimum Viable Products make it to production. Infrastructure, governance, security, compliance and—most critically—end-to-end integration take time.
In this context, adopting AI effectively means navigating three key tensions:
1.Hype and urgency –The pressure to act instantly often outweighs thoughtful evaluation and execution.
2.Low maturity of new technologies –Standards are lacking, methods evolve rapidly and today’s solution may require complete rework tomorrow.
3.Overpromised, underdelivered tools –Many flashy solutions are built for attention or funding, not for enterprise-grade complexity.
This article offers a practical deep dive into these tensions—and how to manage them for real-world success.
From Chaos to Clarity: My AI JourneyWe were early adopters of AI and Large Language Model (LLM) integrations. Not just because we saw high-impact use cases, but also due to a desire to stay relevant amid growing urgency and dramatic forecasts from major consultancies.
In a span of weeks, our focus swung from customer-facing solutions to invoice automation, then to developer
Only after committing to one strategic project did we gain traction. But even then, innovation outpaced stability. For example, during the GPT-3 phase, we spent significant time engineering prompts to generate structured outputs. These prompts were long, token-intensive and unreliable for downstream processing. To manage token limits, we built complex workarounds just to pass information into the model.
Then GPT-4 arrived with native function calling and structured JSON output. It was a game changer, but also a hard reset: much of our previous effort was now obsolete. That’s the reality of early adoption. Some bets pay off, others don’t.
We also explored model fine-tuning and switching providers in search of better performance or lower cost. Many of these efforts had to be rolled back when they didn’t scale or integrate cleanly.
Tools That Shine in Demos, Struggle in RealityIn our pursuit to move faster and scale without extra headcount, we explored emerging no-code and plug-and-play AI tools. The appeal was obvious: empower operations without needing developers. And for very simple tasks, like moving and tagging files, they worked great.
But business value isn’t found in those examples. The moment we introduced real-world needs like authenticating users, managing multiple environments, handling exceptions, those tools hit a wall. Their architecture wasn’t built for enterprise integration, security, or complex, proprietary workflows.
This is the trap of hype: what looks like magic in a demo often falls apart in deployment.
So, Is It Worth Joining the Rat Race?After all this, I asked myself: Should we keep chasing every new development? Or is it wiser to wait for maturity and proven solutions?
The answer, as always, is: it depends. But here’s what helped us cut through the noise:
Focus on Core Value—Not FeaturesBuild around clear, meaningful use cases. Focus on well-known processes with defined business value. They might not be flashy, but they increase your chance of success.
• Start simple.Go live with a basic version before adding complex features. If you can cover 80% of the use cases, defer edge cases to future releases.
• Ignore the buzz.A new model isn’t always better. If your current solution works and is cost-efficient, don’t switch just for novelty.
• Beware of syntactic sugar.Not every “advanced” capability adds value. Agentic reasoning, for example, sounds impressive, but if your process is simple and defined, a straightforward prompt works just as well.
• Evaluate new tools pragmatically.If they don’t enhance your core business value, skip them. Revisit later if needed.
Cut Through the Noise with KnowledgeTo evaluate emerging tools and models, a deep understanding of AI and LLM fundamentals is crucial. Leaders don’t need to code, but they must ask the right questions.
AI fluency is becoming a core business skill. Just as a CFO must understand a balance sheet, today’s CIO must grasp the basics of RAG, model fine-tuning, agent orchestration and deployment architecture.
Without this knowledge, it’s hard to tell what’s transformative versus what’s just good marketing.
Build a New Leadership Muscle: Managing AIManaging AI systems and agents will soon be as vital as managing people. And like any leadership skill, it comes through experience—not delegation.
Think back to the beginning of your career. You didn’t start by leading teams. You started by doing the work. Whether analyzing data or configuring systems, you built the foundation that shaped your leadership style.
The same applies here. Get hands-on. Deploy tools. Learn workflows. Understand limitations. Fail small. Learn fast.
That hands-on experience sharpens your judgment, accelerates your strategic decision-making and earns respect from your teams.
Conclusion: Don’t Run the RaceThe AI race isn’t about who implements the most tools or jumps on the latest trend. It’s about building lasting value with clarity and intent.
Yes, stay curious. Yes, explore. But don’t run blindly. The real differentiator is, knowing when to sprint, when to walk, And when to pause and think.
Weekly Brief
I agree We use cookies on this website to enhance your user experience. By clicking any link on this page you are giving your consent for us to set cookies. More info
Read Also
Streamlining Operations and Empowering Teams in Facilities Management
Technocreativity: The Synergy Of Technology And Creativity
Leading It And Digital Transformation At Ikea: Insights From An Industry Veteran
Executive Leadership And Digital Transformation In The Global Fashion Industry
Digital Transformation in Fashion Retail - From Efficiency to Experience
Driving IT Transformation at Lactalis Australia
AI Adoption in Hospitality: Striking the Balance Between Innovation, Excellence and Trust
The AI Rat Race - Keeping Up with New Technologies or Waiting for Maturity?
